Design recommendation module
- Each user likes a set of movies
- u1 = {m3, m5, m7, m11}
- u2 = {m1, m2, m3, m4, m5, m6, m7, m8, m9}
- Similarity(u1, u2) = 3
- For a user, find his/her top-1 similar user
Remember SNAKE principle
Scenario: Interface
class Recommender {
def findSimilarUser(userId: String)
}
Be aware your naming
Necessary: Constrain/Hypothesis
- Predict
- Max peak users = 1,250,000
- Calculation frequency = 1/10min/user
- Peak QPS (Queries Per Second) =
- Max peak users / Calcuation frequency
= 1,250,000 / (10 * 60)
= 2083/s
- Max peak users / Calcuation frequency
Algorithm: Collaborative Filtering
|
M1 |
M2 |
M3 |
M4 |
M5 |
M6 |
M7 |
M8 |
M9 |
U1 |
V |
|
V |
|
|
|
V |
|
|
U2 |
|
|
V |
|
V |
|
|
|
|
U3 |
V |
|
V |
V |
|
|
|
|
V |
For U1 and U2:
- M1 != M3
- M1 != M5
- M3 == M3
- M3 != M5
- M7 != M3
- M7 != M5
Similarity(U1, U2) = 1
For U1 and U3:
- M1 == M1
- M1 != M3
- M1 != M4
- M1 != M9
- M3 != M1
- M3 == M3
- M3 != M4
- M3 != M9
- M7 != M1
- M7 != M3
- M7 != M4
- M7 != M9
Similarity(U1, U3) = 2
To figure out U1 and others simiarity, it need to perform 18 times calculation.
Roughly performance estimation = 0.2s/query (in general, 10^6 ~ 10^9 can be done within 1 sec, but it depends on the complexity)
Max capability = 5 qps
System design (v1)
Interviewer: How to improve the performance?
Improve performance with inverted index. Previously, we have looked from User to Movie, then now revert the order to look from Moive to User
U1 |
U2 |
U3 |
|
M1 |
V |
|
V |
M2 |
|
|
|
M3 |
V |
V |
V |
M4 |
|
|
V |
M5 |
|
V |
|
M6 |
|
|
|
M7 |
V |
|
|
M8 |
|
|
|
M9 |
|
|
V |
For movie liked by U1: M1, M3, M7
For M1:
- Similarity(U1, U3) += 1
For M3:
- Similarity(U1, U2) += 1
- Similarity(U1, U3) += 1
For M7:
- None
Similarity(U1, U2) = 1
Similarity(U1, U3) = 2
Performance = 0.02s/query
Max capability = 50 qps
System Design (v2)
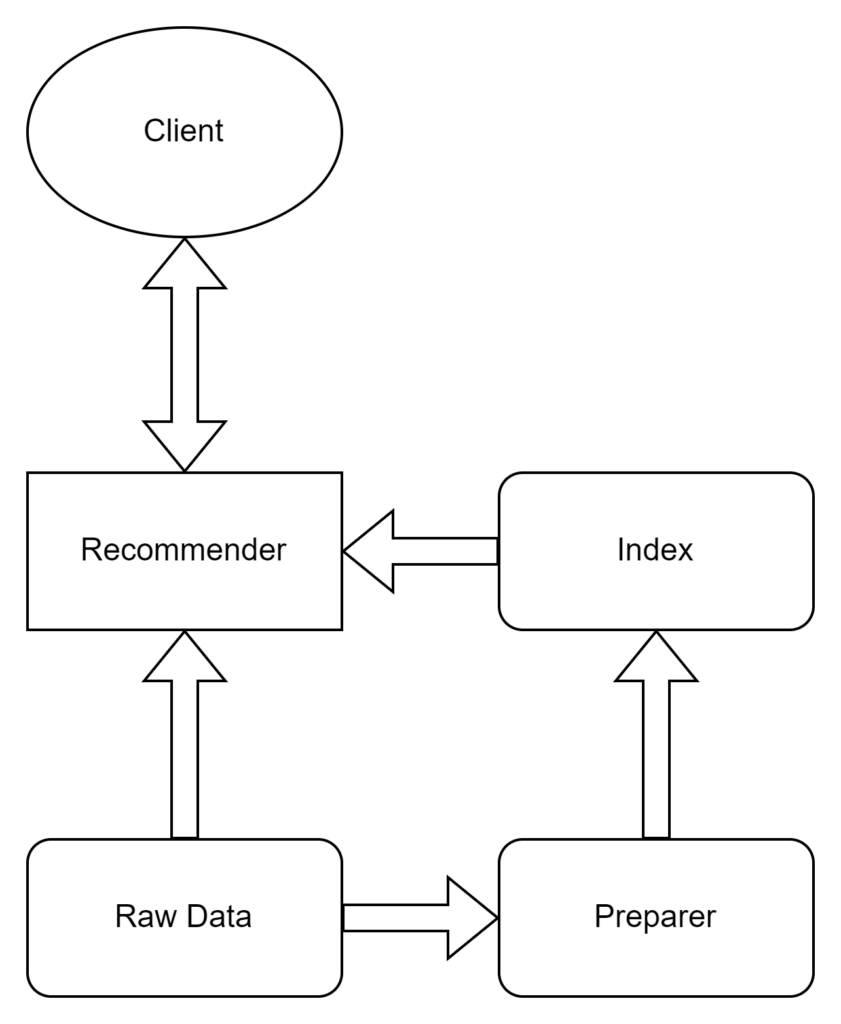
System Design (v3)
In v2, we can estimate the performance is 50 qps, then we can use multiple machine to achieve 2083 qps.
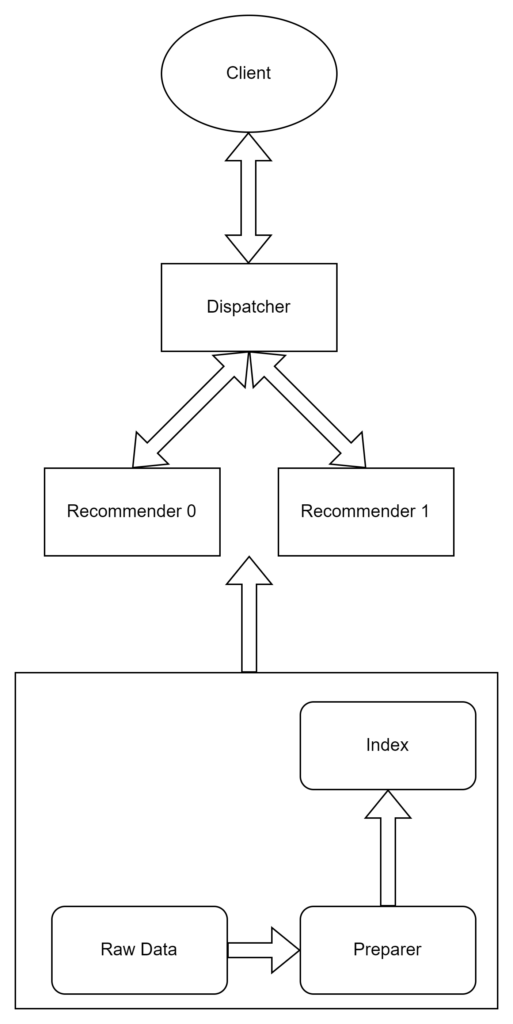
2083 / 50 = 42. Therefore, we need 42 recommenders to achieve 2083 qps.
System Design (v4) with more machines
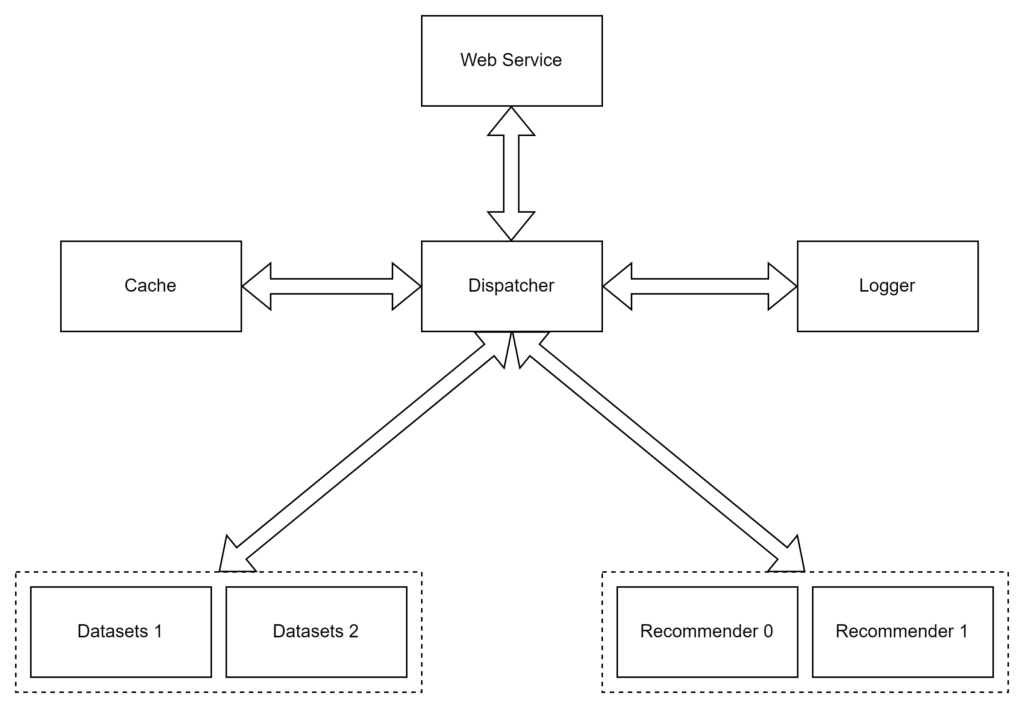
搶先發佈留言